Predictive Maintenance Forum
26-28 April | Online | 8 Tracks | 5 Speakers
5 May | Hands-on Physical Workshop - Singapore
The event is free but registration is required.
Ready? Game On!
GMT +8 | Singapore time
Preventive today, Predictive tomorrow
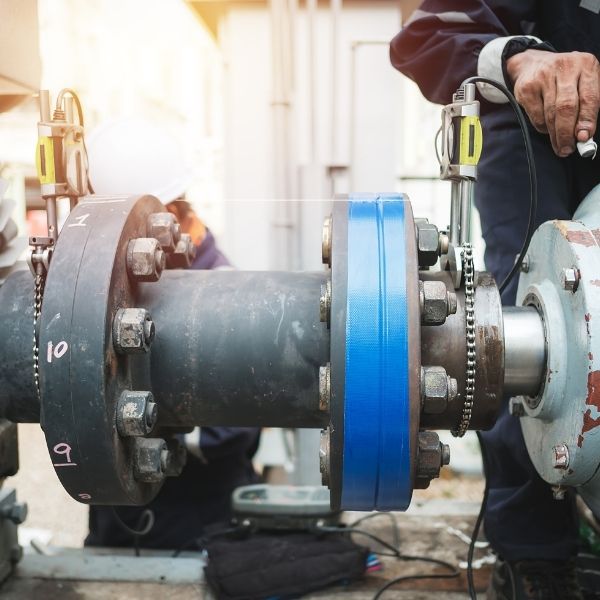
Speakers
Learn and network together
Dr Vineet Jacob Kuruvilla
Industry Manager MathWorks Inc.Dr Vineet is the Aerospace and Defense Industry Manager at MathWorks. He also leads the strategy development and execution for the Predictive Maintenance segment in the AeroDef industry.
Ian M. Alferez
Application Engineering Manager TechSource SystemsIan specializes in in the field of embedded system (embedded coder configuration), data analytics (Machine Learning) and technical computing with MATLAB/Simulink.
Dr Seow Kok Huei
Senior Application Engineer TechSource SystemsDr Seow Kok Huei is a Senior Application Engineer at TechSource Systems, specializing in the application of data engineering, bioinformatics, Image Processing, Deep Learning, and Machine learning to biological datasets for diagnostics.
Claudine Casipit Papag
Application Engineer TechSource SystemsClaudine C. Papag is an Application Engineer at TechSource Systems. She specialized in modeling and simulation, Verification, Code generation, and tool automation with MATLAB/Simulink/Stateflow and cross software configuration.
Agenda
Keynote
Preventive today, Predictive tomorrow
How Industry 4.0 is changing our way of developing..
The industrial world is rapidly changing with the emergence of Industry 4.0, which encompasses the growing complexity of software and an ever-increasing amount of data.
The increasing code base on industrial systems is a challenge for classically trained engineers who rely on traditional methods for programming and testing. Also, sensors on modern equipment collect a significant amount of measured data, which needs to be analyzed to gain knowledge about product quality, energy consumption, machine health status and other economically relevant parameters. With the exponential increase in operational data, AI has become a valuable tool to automate the processing of these data and derive actionable insights, for example, for predictive maintenance.
These two trends will challenge engineers to become proficient in using new methods to emerge from the transformation as leaders in their areas and with new business models for their market.
Introduction to Predictive Maintenance
Learn how predictive maintenance lets you estimate the optimum time to do maintenance by predicting time to failure of a machine. This way, you can minimize downtime and maximize equipment lifetime. In this technical forum, you’ll learn how predictive maintenance works and how it is different from other strategies such as reactive and preventive maintenance. We will also walk you through a workflow that will help you develop a predictive maintenance algorithm.
You’ll learn about condition indicators and how you can extract them from your data to discriminate between healthy and faulty states. Machine learning models are trained using the extracted condition indicators to classify different types of faults. We will also help you understand different estimator models, such as survival, similarity, and degradation, that are used to estimate the remaining useful life of a machine.
Highlights:
Demo Showcases
• Triplex Oil Pump Demo
• Jet Engine Demo-walk through the predictive maintenance workflow and identify conditieon indicators.
**Actual timing may differ on actual day of event
Predictive Maintenance and AI Techniques
Deploying Artificial Intelligence on a PLC (Predictive maintenance algorithm)
Predictive maintenance is increasingly being adopted, as it can reduce unplanned downtimes and maintenance costs when industrial equipment breaks. In this technical forum series, you will see how you can use simulation models of industrial systems along with Model-Based Design to cover the entire predictive maintenance workflow. The workflow spans from data acquisition and preprocessing to design and deployment of the predictive maintenance algorithm onto a PLC and as standalone executable or web application.
Highlights:
• Learn the fundamental aspects of predictive maintenance.
• Learn how physical modeling can help you generate synthetic failure data necessary for the development of your predictive maintenance algorithm.
• See how the Classification Learner app enables you to train and validate your condition monitoring algorithm.
• Learn how to automatically generate code from your machine learning model and test it on real-time hardware (e.g., on a B&R PLC).
• Learn how to build a model to predict the remaining useful life (RUL) of your system.
Deep Learning for Predictive Maintenance
Predictive maintenance allows equipment operators and manufacturers to assess the condition of machines, diagnose faults, and estimate time to failure. Because machines are increasingly complex and generate large amounts of data, many engineers are exploring deep learning approaches to achieve the best predictive results.
• Anomaly detection of industrial equipment using vibration data
• Condition monitoring of an air compressor using audio data
Highlights:
• Explore deep learning approaches to predictive maintenance by detecting anomalies and identifying faults in industrial equipment sensor data.Demo Showcases
Developing a Digital Twin for RUL Estimation A Battery Degradation Example
For this session will run through battery modeling and parameterization, SOC and SOH estimation and lastly determination of its remaining useful life through predictive maintenance algorithm
Highlights:
You will be able to learn:
• Model a battery using Physical Networks• Update Battery Model Parameters (SOC/SOH) during Operation
• Data driven approach to estimate RUL
Predictive Maintenance Hands-on Workshop
- Limited seats for 30 pax Only
Predictive Maintenance Hands-on Workshop (In-person)
- Limited seats for 15 pax Only
This workshop is suitable for anyone who are interested in learning about the workflow for developing PdM algorithms with MATLAB and Simulink.
In this hands-on workshop, you will be able to learn:
• Generating data from Simulink
• Data pre-processing and feature extraction
• Implementing feature extraction
• Predictive modeling
*Terms and Conditions:
• Due to the limited availability of seats, early registration is strongly recommended to ensure your participation.
• Registration will be confirmed upon your receipt of the confirmation, on a first-come-first-serve basis.
• Should you wish to cancel your registration, please inform us in writing to events@techsource-asia.com
• TechSource reserves the right to reschedule or cancel the event due to unforeseen circumstances. Every effort, however, will be made to inform participants of the change.
**Actual timing may differ on actual day of event
Location
Online via Zoom
Physical Workshop @ Singapore Office will be conducted on 5th of May
Top Questions for Predictive Maintenance
Tell us your burning questions for Predictive Maintenance or MATLAB, we will answer you one-by-one!
Why are we interested in Predictive Maintenance?
Costs reduction, Performance Improvement, or do you want to provide a condition monitoring / predictive maintenance service to users of your product?
...But Deploying a Predictive Maintenance Algorithm Successfully Is Much More Complicated
We hear you...
The Challenges Associated With Predictive Maintenance Are Consistent Across Industries, for both Data Scientists & Engineers
We will discuss more during the Forum........................
Developing A Predictive Maintenance Algorithm Requires Domain Expertise and Machine Learning Techniques...
We hear you... Let's discuss more
- Explore and automate feature extraction & machine learning tasks using MATLAB Apps
- Visualize Data, Try Different Feature Extraction Methods & Compare Results Without Writing Any MATLAB Code........................
How are MathWorks Tools Used for Predictive Maintenance?
We will show you Who, How, When through case studies, demos, etc. And if you are lucky enough to get a seat for our hands-on workshop, experience yourself and learn more.